Summary
In many settings, expert professionals like physicians and judges make different decisions when handling similar cases. These differences are often attributed to (inefficient) variation in decision-maker preferences, sparking calls to standardize decision-making. A recent study proposes an alternative framework where experts differ not only in preferences but also in diagnostic skill, with both factors shaping their decisions.
The study applies this framework to pneumonia diagnoses by radiologists, whose diagnosis rates vary widely. The findings suggest that radiologists regard missing a diagnosis as more costly than incorrectly treating a healthy patient. This prompts less-skilled radiologists to diagnose more patients as an optimal response. The study finds that skill variation explains a significant portion of the observed differences in decisions. Imposing uniform diagnosis rates could make outcomes worse overall, whereas improving diagnostic accuracy would yield major welfare gains.
Across many settings, expert decision-makers vary substantially in the way they treat similar cases. This variation has raised widespread concern among policymakers. Standard frameworks for studying variation in decisions assume that all decision-makers observe the same information about the cases before them. Differences in decisions must therefore be due to differences in preferences.
The research summarized here considers an alternative framework in which experts may also differ in their information. We consider a process that involves two steps. First, experts assess the likely outcomes of possible choices, with the accuracy of this assessment reflecting their diagnostic skill. Second, experts weigh the costs and benefits associated with each choice, applying their preferences to reach a final decision.
Distinguishing whether variation in decisions arises from differences in skill or in preferences is crucial for effective policy design. If variation mainly reflects experts’ preferences, reducing the variation in decisions, by aligning preferences with the true social costs of false negatives and false positives, could improve overall outcomes. Conversely, if skill differences are the primary driver, reducing variation in decision rates may prove counterproductive.
While previous studies often attribute decision differences solely to preferences, we show that skill variation can play a large role. The study uses data from the Veterans Health Administration on 5.5 million chest X-rays conducted in emergency departments. We examine radiologists’ pneumonia diagnoses by comparing their diagnosis rates — the percentage of patients diagnosed with pneumonia — and miss rates — the percentage of undiagnosed patients who later receive a pneumonia diagnosis.
We find substantial variation in diagnosis rates among radiologists. If all radiologists had the same level of skill, we would expect to see that those diagnosing more patients would exhibit lower miss rates, since they leave undiagnosed only those who are least likely to have pneumonia. However, we show that radiologists with higher diagnosis rates tend to have higher miss rates in the data we study. This is hard to explain if radiologists are equally skilled, but it makes sense if skills vary substantially and those with less skill optimally choose to diagnose the most patients.
Motivated by these findings, we propose a framework where experts differ in both skill and preferences. Our framework explains the positive relationship observed between diagnosis and miss rates: when missing a pneumonia diagnosis is seen as highly costly, less skilled radiologists choose to diagnose more patients, increasing their diagnosis rates. But due to lower skill, they also tend to miss more cases, resulting in higher miss rates.
The paper shows that, if patients are randomly assigned across radiologists, we can combine information on diagnosis and miss rates to rank radiologists by skill. Estimating a model under additional assumptions, the study finds considerable variation in diagnostic accuracy, with skill differences accounting for almost two-fifths of the observed variation in decisions.
Finally, the paper examines two ways to intervene. Standardizing diagnosis rates could lead to worse outcomes: if radiologists with noisier diagnostic information optimally diagnose more patients, enforcing uniform rates may worsen outcomes overall. In contrast, improving diagnostic accuracy offers the potential for welfare gains.
Main article
Across a wide range of contexts, professionals faced with similar situations often make systematically different choices. For example, physicians may vary in their willingness to prescribe aggressive treatments or to order expensive tests for patients with similar characteristics. Judges differ in their tendencies to impose strict or lenient sentences on comparable defendants. Similar patterns are also observed among teachers, managers, and police officers. This variation is of particular interest to policymakers. The variation may suggest unequal resource allocation across similar cases, often leading to proposals for guidelines to standardize decision-making in order to reduce the variation in decision rates across decision-makers.
When making these decisions, the typical expert will begin by assessing the likely effects of their options based on the specific case at hand. For instance, physicians aim to diagnose a patient’s underlying condition and evaluate potential treatment outcomes, and judges work to determine the facts of a crime and the likelihood of recidivism. The accuracy of these assessments reflects an expert’s diagnostic skill. Following this evaluation, agents weigh the costs and benefits associated with each choice, balancing trade-offs to reach a decision. For example, a physician may consider missing a diagnosis as more costly than incorrectly treating a healthy patient, and a judge might find convicting an innocent person far more objectionable than the possibility of recidivism. These weights reflect the expert’s preferences.
“Our recent study highlights substantial skill differences among radiologists in the context of pneumonia diagnoses”
The systematic variation in decisions may stem from differences in skill, preferences, or both. Identifying where the variation comes from is crucial for evaluating its net costs and benefits, and hence in designing effective policies. If decision variation is primarily driven by differences in preferences, it suggests inefficiency relative to how an overarching “social planner” would approach the problem. A social planner would weigh the costs of false negatives and false positives in the interests of society as a whole. Then, standardizing decision rates could improve outcomes. Conversely, if the variation arises mainly from differences in skill, it does not necessarily imply inefficiency, and reducing the variation in decision rates might even be counterproductive.
Variability in pneumonia diagnoses
Many previous studies have focused solely on differences in preferences, often neglecting the role of skill variation. Our recent study highlights substantial skill differences among radiologists in the context of pneumonia diagnoses, emphasizing the importance of considering both skill and preference variation. Pneumonia affects hundreds of millions of people and causes millions of deaths worldwide each year. Despite the availability of antibiotic treatment, it remains one of the leading causes of death in the United States.
The paper analyzes data from the Veterans Health Administration on 5.5 million chest X-rays conducted in emergency departments (EDs). Radiologists are assigned to imaging cases nearly at random at each location and time. Each radiologist must decide whether to diagnose a patient with pneumonia and initiate antibiotic treatment based on the X-rays and other available information. The proportion of patients diagnosed with pneumonia by a radiologist is referred to as their diagnosis rate. For patients who are not diagnosed in the ED, some may return later and receive a pneumonia diagnosis within a few days. The proportion of a radiologist’s patients who leave the ED with undiagnosed pneumonia is referred to as their miss rate.
Using the data, the study first documents significant variation in diagnosis rates among radiologists. For example, reassigning patients from a radiologist in the 10th percentile of diagnosis rates to one in the 90th percentile would increase the likelihood of a diagnosis from 8.9% to 12.3%. This finding is consistent with previous evidence showing substantial variability in pneumonia diagnoses across and within radiologists, even when interpreting the same chest X-rays.
“After accounting for location and time, radiologists with higher diagnosis rates exhibit higher — rather than lower — miss rates on average”
If all radiologists had equal diagnostic skill, the observed variation in diagnoses would arise solely from differences in preferences. In such a scenario, with cases randomly assigned to radiologists, those who diagnose more patients should have lower miss rates on average, as they would be exercising greater caution to avoid missed diagnoses. However, as illustrated in Figure 1, after accounting for location and time, radiologists with higher diagnosis rates exhibit higher — rather than lower — miss rates on average. This implies that a patient randomly assigned to a radiologist with a higher diagnosis rate is more likely to leave the ED with undiagnosed pneumonia compared to one seen by a radiologist with a lower diagnosis rate.
Figure 1: Diagnosis and Miss Rates
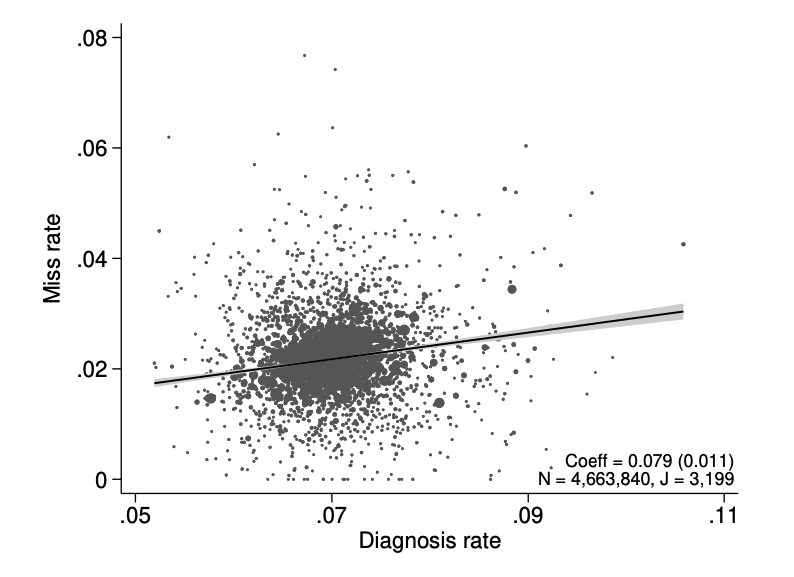
Figure 1 also reveals that miss rates vary widely even when diagnosis rates are held constant. For the same diagnosis rate, a radiologist in the 90th percentile of miss rates has a miss rate 0.7 percentage points higher than one in the 10th percentile. This evidence challenges the hypothesis of uniform skill among radiologists, suggesting that skill differences play a significant role in these decisions.
This finding matters for policy. Efforts to standardize diagnosis rates could overlook meaningful improvements in skill, potentially missing opportunities to raise diagnostic accuracy. Furthermore, standardization policies could be counterproductive if differences in diagnostic skill make varying diagnosis rates optimal. For instance, if missing a diagnosis is more costly than falsely diagnosing a healthy patient, a radiologist with noisier diagnostic information may optimally diagnose more patients. Requiring him or her to do otherwise could inadvertently reduce efficiency.
A skill-preference framework
To explain these empirical observations, the paper proposes a framework in which agents differ in both skill and preferences, with their decisions shaped by these two factors jointly. The framework begins with a “classification problem” where both decisions and underlying “states of the world” are binary (that is, there are only two possibilities in each case). In this context, the decision is whether to diagnose a patient and initiate antibiotic treatment, while the underlying state is whether the patient has pneumonia. A radiologist first examines chest X-rays and other relevant information to form a signal regarding the likelihood of disease, ranking cases by their suitability for treatment. She then chooses a threshold within this ranking, diagnosing cases with signals above this threshold. In selecting this threshold, the radiologist weighs the relative costs of a false negative (i.e., missing a diagnosis) versus a false positive (i.e., mistakenly diagnosing a healthy patient).
“if missing a diagnosis is more costly than falsely diagnosing a healthy patient, a radiologist with noisier diagnostic information may optimally diagnose more patients”
In this framework, skill refers to the correlation between the signal a radiologist forms and the patient’s disease state; the higher the skill, the more accurate the signal in reflecting the presence of pneumonia. Preference, on the other hand, refers to the radiologist’s weighting of the costs associated with different diagnostic errors — specifically, the relative importance of a false negative and a false positive.
This framework can explain the positive relationship observed between diagnosis and miss rates. If missing a pneumonia diagnosis is considered more costly than falsely diagnosing a healthy patient, a radiologist with lower diagnostic skill will rationally choose to diagnose more patients. Seeking to mitigate the risk of missed cases results in a higher diagnosis rate. However, this less skilled radiologist may also make more errors, leaving more patients with undiagnosed pneumonia and resulting in a higher miss rate.
Unpacking skill and preferences
The paper shows that when patients are randomly assigned to radiologists, information on diagnosis rates and miss rates can be combined to identify their relative skill levels with minimal assumptions. For any pair of radiologists, if one has both a higher diagnosis rate and a higher miss rate than the other, that radiologist must be of lower skill. Similarly, if a radiologist has a lower or equal diagnosis rate but a significantly higher miss rate — one that exceeds any differences in diagnosis rates — this radiologist must also be of lower skill.
A common tool for summarizing classification accuracy is the receiver operating characteristic (ROC) curve. It plots the true positive rate (TPR) — the share of true positives among all positive cases — against the false positive rate (FPR) — the share of false positives among all negative cases. Ideally, TPR=1 (no missed diagnoses) and FPR=0 (no unnecessary diagnoses). In our model, an agent’s ROC curve defines the boundary of achievable classification outcomes as she adjusts the proportion of cases classified as positive. If the agent diagnoses no cases, TPR and FPR are both 0; if she diagnoses all cases, both are 1. As the diagnosis rate increases, TPR and FPR also increase. The ROC curve thus resembles what economists call a “production possibility frontier”, but in this case for “true positive” and “true negative” diagnoses, where higher curves indicate greater skill in the evaluation stage. An agent’s position on her ROC curve reflects her preferences.
Figure 2: Projecting Data on ROC Space
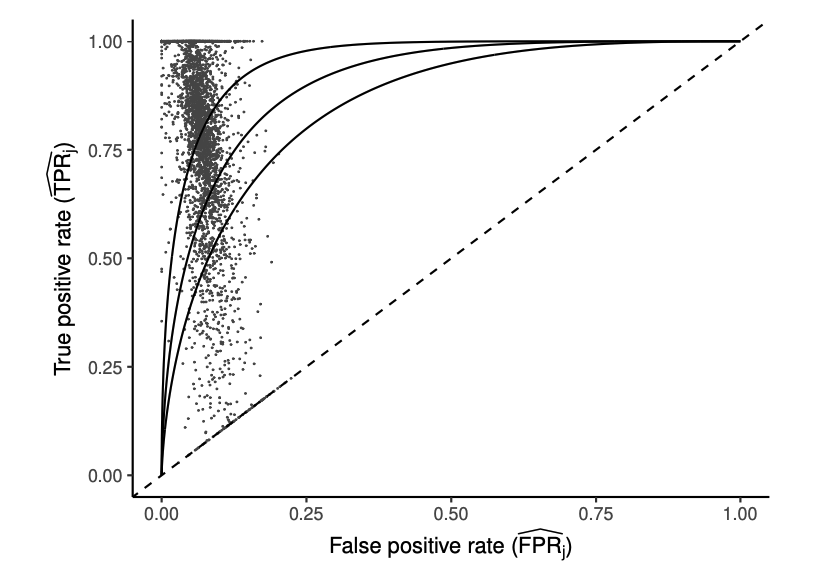
Figure 2 maps each radiologist’s observed diagnosis and miss rates onto TPR and FPR based on model estimates. Solid lines represent ROC curves at different skill levels, with each point corresponding to a radiologist. The spread of points shows that radiologists do not lie along a single ROC curve, indicating that skill varies substantially.
The results assume that cases are randomly assigned to radiologists, for any given location and time. If this assumption is wrong, and one radiologist is assigned sicker patients — those who are inherently more likely to have pneumonia — compared to another radiologist working at the same location and time, the former may exhibit both a higher diagnosis rate and a higher miss rate, even with identical skill levels.
Assuming random assignment is plausible in the ED setting, due to the idiosyncratic variation in patient arrivals and in the availability of radiologists at specific times and locations. We draw on the dataset to support this: we show that a comprehensive set of patient characteristics and clinical information, which are strongly associated with underlying conditions, has limited predictive power for radiologist assignment. This implies that the pool of patients seen by each radiologist has a similar average risk of having pneumonia. Furthermore, the results remain robust when these patient characteristics and clinical data are either controlled for, or excluded in the analysis.
“when patients are randomly assigned to radiologists, information on diagnosis rates and miss rates can be combined to identify their relative skill levels with minimal assumptions”
With additional assumptions about the structure of the signals that radiologists receive regarding patient conditions, their “utility functions” (preferences), and the joint distribution of skill and preferences, the heterogeneity in radiologists’ skill and preferences can be separately identified from the data. The estimation results indicate that, on average, a radiologist’s diagnostic signal has a correlation of 0.85 with the patient’s underlying state. However, diagnostic accuracy varies widely among radiologists, with correlations ranging from 0.76 in the 10th percentile to 0.93 in the 90th percentile. On average, radiologists view missing a diagnosis as 6.71 times more costly than making an unnecessary diagnosis; this ratio varies from 5.60 to 7.91 between the 10th and 90th percentiles. Overall, eliminating variation in skill would reduce variation in diagnosis rates by 39% and variation in miss rates by 78%. In contrast, eliminating variation in preferences would reduce variation in diagnosis rates by 29% and has no significant effect on variation in miss rates.
Diagnostic skill also varies across different groups of radiologists. Older radiologists and those assessing higher volumes of chest X-rays generally have better diagnostic skill. Interestingly, higher-skilled radiologists tend to produce shorter reports of their findings, but spend more time generating those reports. This suggests that effort — rather than raw talent alone — may contribute to radiologist skill.
What this implies for policy
Using the model estimates, the paper examines two types of policy interventions. From society’s point of view, the considerations include both false negatives (missed diagnoses) and false positives (unnecessary diagnoses), evaluated through an objective function that reflects the preferences of an overall social planner, assumed to align with those of the average radiologist.
The first policy imposes a fixed diagnostic threshold aimed at maximizing welfare under the (incorrect) assumption that all radiologists have equal skill. While this approach eliminates diagnostic variation, simulations show that a fixed threshold would lead to worse outcomes. Although it reduces unnecessary diagnoses, it leads to an increase in missed diagnoses, which are more costly. The rationale behind this result is that lower-skilled radiologists tend to diagnose more patients to mitigate missed diagnoses. Forcing them to adhere to a fixed threshold restricts this adaptation and can be counterproductive.
A more flexible approach involves setting thresholds that vary optimally according to each radiologist’s skill level. By design, this policy enhances welfare. However, the potential welfare gains are modest. Also, since skill levels are often unobserved in practice, implementing such a policy would be difficult.
“Improving diagnostic skill leads to significant reductions in both missed and unnecessary diagnoses, resulting in a major welfare gain — outperforming any threshold-based policy”
The second policy focuses on enhancing diagnostic skill itself, which might be achieved through training radiologists differently, selecting those with higher skill levels, or aggregating signals to improve information in decision-making. Specifically, we consider a policy that raises the skill level of radiologists in the bottom quartile of the distribution up to the 25th percentile, while continuing to allow radiologists to make decisions based on their own preferences. Simulations indicate that improving diagnostic skill leads to significant reductions in both missed and unnecessary diagnoses, resulting in a major welfare gain — outperforming any threshold-based policy.
Through these simulations, the paper emphasizes that “skill” and “preferences” are distinct in what they imply for welfare and policy. In practice, each dimension likely reflects various underlying factors, and their relative importance can vary significantly depending on the context. For example, “skill” may reflect not only a radiologist’s inherent ability but also the effort they exert. Radiologists often make judgments with input from others, such as residents, fellows, and non-radiologist clinicians, and communicate their findings to other physicians. Thus, skill could also reflect the quality of information conveyed by the radiologist or their team. Meanwhile, “preferences” here represent deviations from the objectives of a social planner, which may arise from intrinsic preferences, individual biases, or external incentives.
Although the specific mechanisms driving “skill” or “preferences” may not directly affect welfare analysis in our study, they will matter for good policy. For instance, deciding whether to invest in radiologists’ training for reading chest X-rays, encouraging collaborative practices, or improving communication skills, will depend on the institutional factors that shape these dimensions.
This article summarizes “Selection with variation in diagnostic skill: Evidence from radiologists” by David C. Chan, Matthew Gentzkow, and Chuan Yu, published in the Quarterly Journal of Economics in May 2022.
David Chan is Associate Professor of Health Policy at the Stanford School of Medicine and an investigator at the Department of Veterans Affairs. Matthew Gentzkow is Landau Professor in Technology and the Economy at Stanford University. Chuan Yu is a Postdoctoral Fellow at Harvard Business School.