Summary
The US labor market is particularly dynamic for Science, Technology, Engineering, and Math (STEM) jobs, with new technologies proliferating throughout workplaces every year. This technological change is the engine of long-run productivity growth, but it also means that workers in technology-intensive occupations must constantly learn on the job, or risk becoming obsolete.
This paper considers the consequences of technological obsolescence for workers in STEM occupations. We have three main findings. First, we look at how job skills change over time by studying the appearance of new skills and the disappearance of old skills over time. The overall rate of skill turnover is high—comparing vacancies posted by the same firm for the same occupation in 2007 and 2019 we find that about 29% contained at least one new skill requirement in 2019. Occupations vary systematically in the amount of skill turnover, however, with STEM and other technology-intensive occupations changing significantly faster than other jobs.
Second, we build a simple model that generates empirical predictions about life-cycle earnings for college graduates making a variety of career choices. The key insight is that life-cycle wage growth will balance gains from on-the-job learning against losses that come from skill obsolescence. Our main prediction is that workers in rapidly changing fields will experience slower wage growth, meaning that high-ability workers will tend to switch from fast-changing to slow-changing careers over time.
Third, we test the predictions of our model by comparing estimates of the economic returns to working in faster- and slower-changing careers. We compute a college major-specific measure of job skill change, and show that the economic returns to majoring in and working in STEM are initially high, but decline by more than half in the first decade of working life. This is not because STEM jobs pay less over time, but because workers in other occupations partially catch up. Unsurprisingly, therefore, we also find that higher-ability college graduates are more likely to exit STEM jobs over time. High-ability college graduates are about 5 percentage points more likely to work in STEM at age 23, but no more likely than workers of average ability to hold a STEM job by age 40.
These findings have important policy implications. At a minimum, our results suggest that college students should be presented with information about earnings trajectories by major, not just earnings at labor market entry. More broadly, colleges and policymakers should be mindful of an important tradeoff; technology-specific skills may make for a smoother entry into the workforce than more general skills, but may also have a shorter shelf life due to rapid technological change. Much of the longer-term value of a bachelor’s degree may therefore come from breadth rather than depth.
Main article
STEM industries are a major engine of long-run productivity growth. This paper finds that skill requirements for STEM jobs turn over much faster than in other industries. This means that workers must constantly learn on the job, or risk becoming obsolete. We show that workers in rapidly changing fields experience slower wage growthāthe economic returns to majoring in and working in STEM are initially high but decline by more than half in the first decade of working life. We also show that high-ability workers, who are fast learners, will tend to switch from these fast-changing careers to slower-changing careers in which learning gains accumulate. These findings have important implications for university curriculum design and studentsā choice of undergraduate major.
The US labor market is particularly dynamic for Science, Technology, Engineering, and Math (STEM) jobs, with new technologies proliferating throughout workplaces every year. This technological change is the engine of long-run productivity growth and rising living standards. In the shorter run, however, workers in technology-intensive occupations must constantly learn on the job, or risk becoming obsolete.
New research finds that STEM workers have a higher rate of skill turnover than workers in other industries
This paper considers the consequences of technological obsolescence for workers in STEM occupations. We have three main findings. First, using data on skill demands from more than a decade of online job vacancies, we show that STEM and other technology-intensive occupations really do change faster than other jobs. Second, we develop a simple framework for thinking about wage growth in careers that vary in their rate of technological change. Our main prediction is that workers in rapidly changing fields will experience slower wage growth, leading to exit from technology-intensive professions over time. Third, using data from the American Community Survey (ACS), we show that the economic returns to majoring in and working in STEM are initially high, but decline by more than half in the first decade of working life. This is not because STEM jobs pay less over time, but because workers in other occupations catch up.
How are skill requirements changing in the economy overall?
We know anecdotally that jobs change over time, but there has been little effort in the past to quantify the impact of technology on job skill requirements. We measure job skill change directly using data from Burning Glass Technologies (BG), an employment analytics and labor market information firm that scrapes job vacancy data for more than 40,000 online job boards and company websites. BG translates key words and phrases from job ads into a large number of unique skill requirements. These range from vague and general (e.g. detail-oriented, problem solving, communication skills) to detailed and job-specific (e.g. phlebotomy, Javascript, truck driving). The data span 2007-2019.
Vacancy data are ideal for measuring the changing skill requirements of jobs, as vacancies directly measure employer demand for specific skills. Because of data limitations, however, most prior work in economics studies changes in demand across occupations (Autor, Levy and Murnane 2003; Deming 2017).
One natural way to measure job skill change is to study the appearance of new skills and the disappearance of old skills over time. We define old skills as those with at least 1000 appearances in 2007 and that either no longer exist or are at most one-fifth as frequent in 2019. Similarly, we define new skills as those with at least 1000 appearances in 2019, and that either did not exist in 2007 or were 20 times more frequent in 2019 compared with 2007. These thresholds are arbitrary, but the results are not sensitive to different choices.
The paper finds that the high rate of skill turnover amongst STEM workers leads to slower wage growth
Figure 1 shows the change in the share of job ads that requested old skills and new skills in 2019 for different types of jobs. The estimates are adjusted for a number of factors, including the specific occupation-city-employer combination of the vacancy. From this figure, there are three main takeaways. First, the overall rate of skill turnover is high. Among vacancies posted by the same firm for the same occupation, about 29% contained at least one new skill requirement in 2019.
Second, occupations vary systematically in the amount of skill turnover. Around 47% of computer and mathematical jobs required at least one new skill in 2019, compared to less than 20% for jobs in fields such as education, law, and community/social services. Other job categories with high rates of skill change include design/media, business, and management.
Third, the jobs that require new skills also have the highest rates of obsolescence. About 16% of computer and mathematical job vacancies in 2007 listed a skill that had become obsolete by 2019. Design/media and business also have relatively high rates of skill obsolescence, while education and healthcare have the lowest.
Figure 1: Turnover of Skill Requirements by Occupation Category
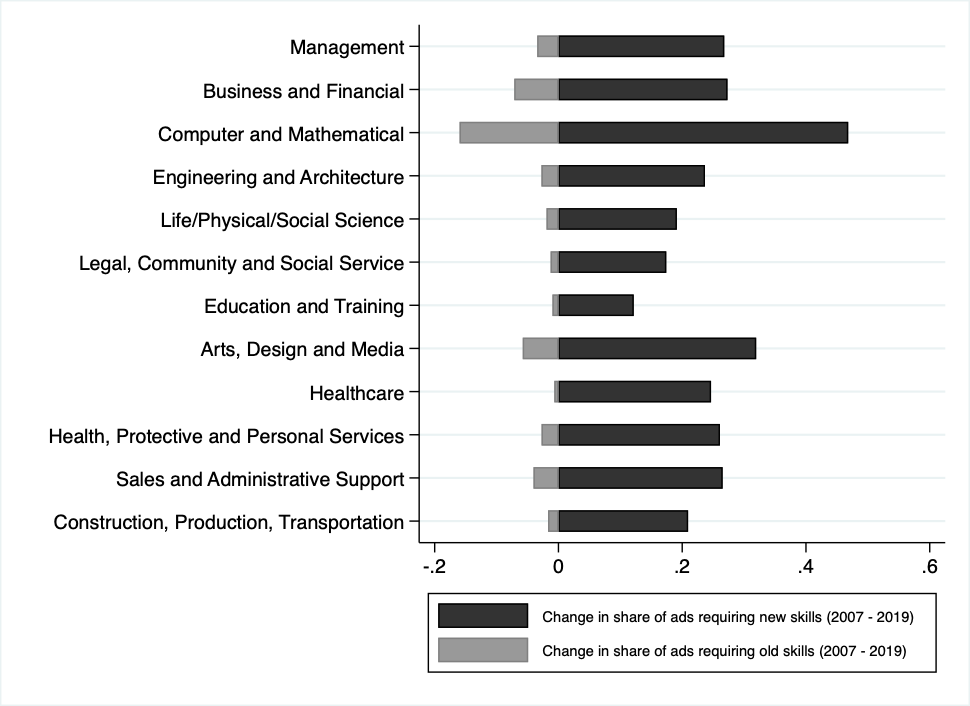
About one third of the turnover in Figure 1 is driven by changes in specific software skill requirements. Because software requirements are specific and verifiable, they are likely to signal other substantive changes in job skills that our data do not fully capture. The fastest-growing software skills between 2007 and 2019 include Python, R, Apache Hadoop, and Revit. Software that was relatively common in 2007, but obsolete by 2019, includes QuarkXpress, Adobe Flash, ActionScript,Solaris, and IBM Websphere.
Measuring changes in the skill content of jobs
We collect all the skill requirements that ever appear in a job vacancy for each occupation, both in 2007 and 2019. We then calculate the share of job ads in which each skill appears in each year and within each occupation. We compute the absolute value of the difference in shares for each skill and then sum them up for each occupation to obtain a job-level measure of overall skill change.
Suppose, for example, that 20% of job vacancy postings for computer scientists required the coding language Python in 2007. This means the occupation ācomputer scienceā would receive a 0.2 for Python in 2007. If the percentage of computer science vacancies requiring Python rose to 80% in 2019, then the absolute difference between 2007 and 2019 for this skill would be 0.6āthe difference between 0.8 and 0.2. Our skill change measure adds these values up for every skill ever listed in each occupation. Conceptually, our measure captures the amount of net skill changeāor turnoverāin an occupation.
By this measure, the jobs with the highest rates of skill change include computer occupations, engineers, scientists and science technicians, business and financial specialists, and managers in technology-intensive fields such as advertising, marketing, and operations. The jobs with the least amount of skill change include drivers, teachers, and food preparation and personal service workers.
Exposure to skill change: the role of college majors
What is the role of education in teaching new, highly in-demand skills? And do certain majors disproportionately funnel graduates into rapidly changing fields?
To answer these questions, we compute a college major-specific measure of job skill change. Specifically, we calculate the average skill change of the occupations held by college graduates in each major when they are between the ages of 22 and 26.
The two majors with the fastest rate of skill change are computer science and engineering, followed by business, communications, and architecture. Computer science and engineering majors rank especially high on this measure, not only because technology-intensive jobs have higher rates of skill change, but also because the distribution of jobs is much more concentrated for these āapplied STEMā majors than for others. Around 58% of computer science majors work in computer-related occupations after graduating, and the corresponding figure for engineers is 42%. In contrast, while life scientist is a fast-changing profession, biology majors take a wide range of jobs, and only a small number actually become scientists. Thus, the rate of job skill change for those majoring in biology is around the sample average. Education majors rank at the bottom because most education majors go into teaching, which is a slow-changing field.
The patterns of career choice across college majors suggest that some college graduatesāparticularly computer science and engineering majorsālearn specific skills that are in high demand, but that are also changing rapidly over time. In the next section we explore the implications of job skill change for life cycle earnings and career trajectories.
The implications of skill change for earnings dynamics
In the paper we build a simple model that generates empirical predictions about life-cycle earnings for college graduates making a variety of career choices. The key insight is that overall life-cycle wage growth balances gains from on-the-job learning against losses that come from skill obsolescence.
In an occupation that is changing rapidly, skills that used to be relevant become obsolete and new skills need to be learned on the job. Each time an old skill is replaced by a new skill, a worker becomes less productive until they can learn how to perform this new skill well.
This means that faster-changing careers have flatter age-earnings profiles. In slow-changing careers, workers continue to get better at the skills required on the job, because they rarely change. In fast-changing careers, workers are always forced to learn new things, and so learning gains cannot accumulate.
Our simple model yields two main predictions:
- Wage growth is lower in careers with higher rates of skill change: Intuitively, careers with high rates of obsolescence require workers to learn many new tasks each year, which diminishes learning gains and lowers the returns to experience.
- High-ability workers will tend to switch from fast-changing to slow-changing careers: As skills become obsolete, the loss associated with leaving a fast-changing career goes down, making it more tempting to switch to slow-changing careers. This is particularly true for high ability workers who can transition across careers and learn more easily.
Declining life-cycle returns to rapidly changing careers
We test the predictions of our model using several different approaches. First, we compare estimates of life-cycle returns to working in faster- and slower-changing careers. We find that majors with a one standard deviation higher rate of skill change (roughly equivalent to the difference between Engineering and History) pay about 50 percent more at age 24, but only 25 percent more at age 34 and only 16 percent more at age 50.
Second, we directly show the economic returns to majors at different ages. Figure 2 shows that the return (in natural log units) to majoring in computer science and engineering relative to all other majors not shown in the Figure is about 0.45 in natural log units in the first few years after college, or 57%. It is only about 0.33 in logs or 39% by age 50. In contrast, both social science and life and physical science majors see their earnings grow in relative terms over the same period. What was initially a very large gap at labor market entry narrows considerably over about a decade.
Figure 2: Relative return for majors
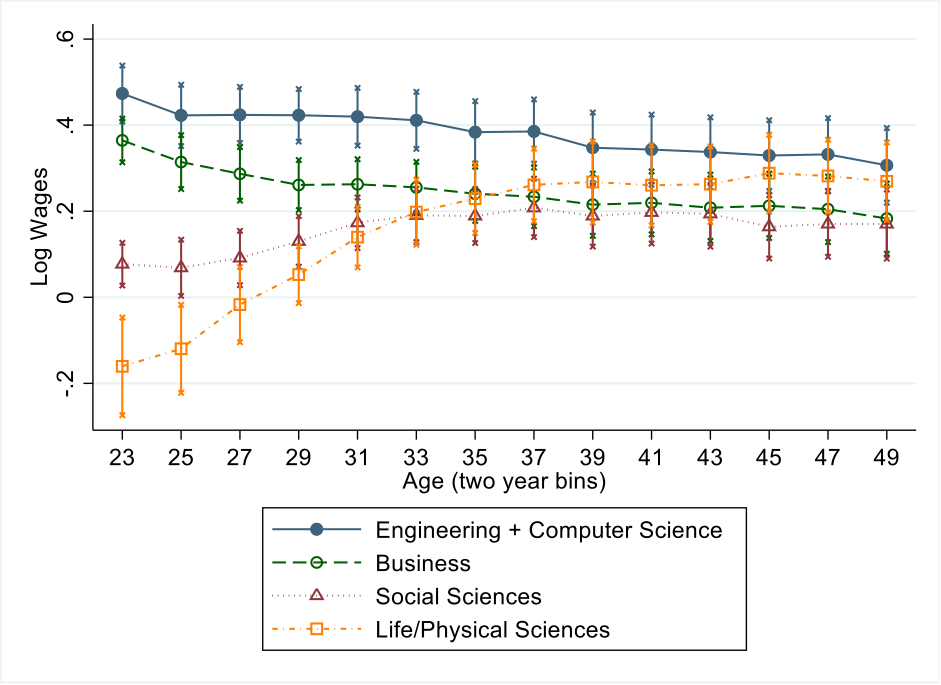
We also find the same pattern of declining returns by age for those working in STEM occupations in three different data sources: the ACS, the Current Population Survey (CPS), and the National Survey of College Graduates (NSCG).
Finally, we explore patterns of occupational sorting over the life cycle. In the ACS, the share of computer science and engineering majors working in computer science and engineering occupations declines from 58% at age 26 to 41% by age 50. Additionally, in the National Longitudinal Survey of Youth (NLSY), we find evidence that higher-ability college graduates are more likely to exit STEM jobs over time. High-ability college graduates are about 5 percentage points more likely to work in STEM at age 23, but no more likely than workers of average ability to hold a STEM job by age 40.
Policy Implications
At a minimum, our results suggest that college students should be presented with information about earnings trajectories by major, not just earnings at labor market entry. More broadly, our findings suggest that much of the value of a bachelorās degree may come from breadth rather than depth, and that āsoft skillsā such as critical thinking and abstract reasoning are a big part of the value of a college education.
Our results also have potentially important implications for public investment in education. The high-skilled vocational preparation provided by STEM degrees paves a smoother transition for college graduates entering the workforce. At the same time, rapid technological change can lead to a short shelf life for technical skills. This tradeoff between technology-specific and general skills is an important consideration for policymakers and colleges seeking to educate the workers of today, while also building the skills of the next generation.
This article summarizes āEarnings Dynamics, Changing Job Skills, and STEM Careersā by David J. Deming and Kadeem Noray, published in The Quarterly Journal of Economics in November 2020.
David J. Deming is the Isabelle and Scott Black Professor of Political Economy at the Harvard Kennedy School, and the faculty director of the Malcolm Wiener Center for Social Policy at Harvard University. Kadeem Noray is at the Harvard Kennedy School and the Department of Economics at Harvard University.